Quick Start on AWS
This example will demonstrate how to automate hyper-parameter search with Milano on AWS.
Before you start
If you have a machine with at least on NVIDIA GPU, it is highly recommended that you try Azkaban-based option first. Please see here for a quick start mini-tutorial.
When using AWS make sure you are aware of all the costs involved.
Once finished, make sure all “milano-worker” instances are termiated! (see screenshot below)
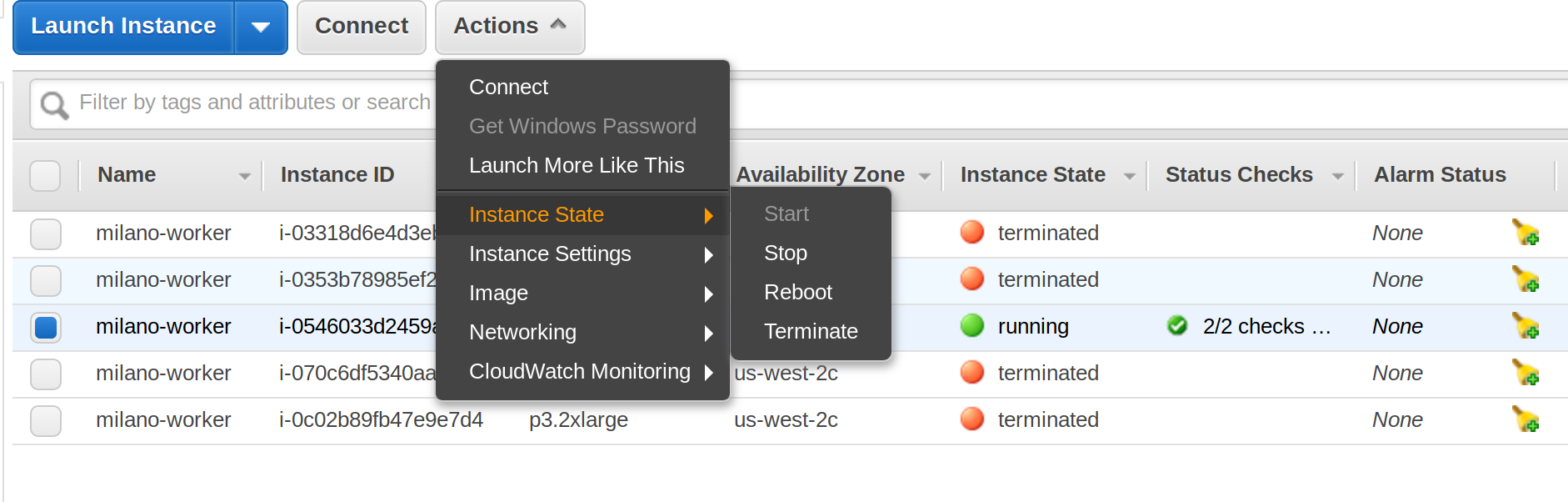
Step 1 (Setup AWS access)
- Registed for AWS account.
- Create a file
~/.aws/credentials
with the following content[default] aws_access_key_id = ..fill this.. aws_secret_access_key = ..fill this..
- To create your
aws_access_key_id
andaws_secret_access_key
, please refer to this AWS document. - You would also need to create a KeyPair in “US-West (Oregon)” region. Go to https://us-west-2.console.aws.amazon.com/ec2 and look for Key Pairs. Once you click “Create Key Pair” .pem file will be downloaded.
Attention: Keep your AWS credentials secret!
Step 2 (Create S3 bucket and upload your data there)
Milano uses S3 buckets for storing training data and saving model checkpoints.
- Install AWS CLI utility:
sudo apt install awscli
(on Ubuntu Linux) - Create S3 bucket:
aws s3api create-bucket --bucket milano-test-data --region us-west-2 --create-bucket-configuration LocationConstraint=us-west-2
- This command created an S3 bucket called ‘milano-test-data’
- Make sure you use ‘us-west-2’ region
- Upload your training data to the bucket you’ve just created. In the steps below we’ll upload CIFAR-10 training data as example:
- Get data:
wget https://www.cs.toronto.edu/~kriz/cifar-10-binary.tar.gz
- Unpack:
tar -xvf cifar-10-binary.tar.gz
- Upload:
aws s3 sync cifar-10-batches-bin/ s3://milano-test-data/cifar-10/cifar-10-batches-bin/
- Get data:
Step 3 (Prepare the job script)
Take a look at these example job scripts:
- “CIFAR-10 classifier model”
examples/os2s/cifar10/start_aws.sh
- This model uses OpenSeq2Seq toolkit which is based on Tensorflow
- Note that by default this model will look for the data under
/data
- “Simple language model”
examples/pytorch/wlm/start_wlm_aws.sh
- This model uses Pytorch and is taken from Pytorch examples repository without any changes
- Note that we use
/workdir
as working directory
For both examples, we set number of epoch to 3 to demonstrate the concept
Step 4 (Prepare the tuning config)
Take a look at these example tuning configs:
- For CIFAR-10 classifier model:
examples/os2s/cifar10/cifar10_aws.py
- Note “datasets” section of the configuration file - this is how we mount and use S3 bucket with our training set
- For Simple language model:
examples/pytorch/wlm/wlm_aws.py
Note that in both cases we set "num_evals": 3
and "num_workers": 1
for illustration purposes.
You might need to contact AWS support to increase the maximum number of P3 instances you can launch at a time.
User predefined parameters
Before running any random search it is a good idea to test several configurations pre-defined by users.
You can do it by including params_to_try_first
section in your config.
For example (from examples/pytorch/wlm/wlm_aws.py
)
# These configurations will be tried first
params_to_try_first = {
"--model": ["LSTM", "GRU"],
"--emsize": [1504, 1504],
"--nlayers": [2, 2],
"--lr": [20, 25],
"--bptt": [35, 35],
"--clip": [0.25, 0.35],
"--dropout": [0.2, 0.2],
}
The above dictionary defines 2 configurations which (one with LSTM and another one with GRU cells) which will be tried before RandomSearch algorithm starts.
Step 5 (Start tuning)
From the client machine run:
“Simple language model”: python tune.py --config=examples/pytorch/wlm/wlm_aws.py --verbose 3
or
“CIFAR-10 classifier model”: python tune.py --config=examples/os2s/cifar10/cifar10_aws.py --verbose 3
Results
By default, the results will be saved in the file results.csv
. They will be ordered with the top results first.
Also, it will be updated on the fly, as results come in.
If a job failed for whatever reason, it will be still logged in results.csv
with inf
as a result.